
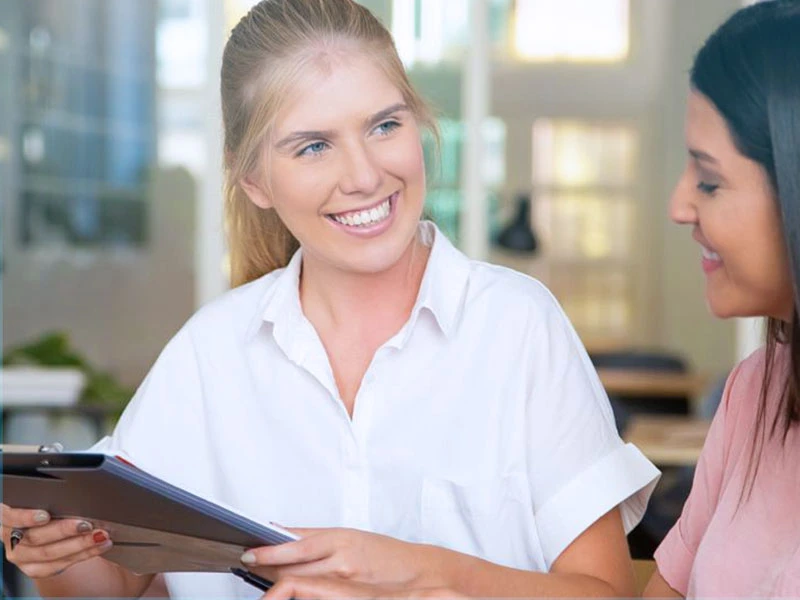
.png)
Real-Life AI Solution for Customer Management
CSS automates categorization of customer feedback
Initial Situation and Challenges
The CSS Group, headquartered in Lucerne, was founded in 1899. Today, the traditional company is one of the leading Swiss health and property insurers. It is the market leader in basic insurance. CSS provides its customers with information offering guidance and support in making decisions on health issues. For years, the insurance company has been collecting around 60,000 customer feedbacks annually at relevant contact points in order to monitor and improve service quality.
The feedbacks are manually labelled in many detailed categories, which involves a lot of work. Jointly with b.telligent, CSS wanted to develop AI methods based on natural language processing to automate the categorization of customer feedback. The sought solution was to be able to handle all aspects of customer feedback. This includes the ability to assign each feedback to multiple categories (multi-label), and furnish each feedback category with a sentiment. The initial situation was highly complex, as customer feedback is recorded in four languages, and varies in length and complexity. Previous attempts at automation were unsuccessful due to the high quality standards of CSS.
Solution Approach
Once b.telligent's team had acquired a sound understanding of the business, the next step was a study and initial analysis of data. This was followed by design of a customized solution for CSS. Specifically, this involved development and implementation of a pipeline which uses selected modern NLP methods for automation. Used here was BERT - a pre-trained, large-language model very similar in architecture to ChatGPT.
Pre-training takes place with a large amount of text data (including the entire encyclopedia Wikipedia and countless newspaper articles), and results in a model with a very good general understanding of language and context. With the help of the manually labelled customer feedback used as training data, the model was re-trained in a fine-tuning step to understand the specific context of health insurance and be able to classify feedback into the right categories with corresponding sentiments. The trained model receives the body text of customer feedback as input parameters, and outputs categories as well as sentiments.
Validation of the model has revealed a very high quality. A review of discrepancies remaining between manual categorization and that by the model has furthermore uncovered inconsistencies in the manual categorization of customer feedback. It turns out that such an AI model achieves more consistent and reproducible quality than a manual approach.
Voices From the Project
b.telligent Services at a Glance
Method development
Development of methods for automated categorization of feedback.
NLP methods
Selection and implementation of modern NLP methods.
Pipeline implementation
Implementation of a pipeline for data preparation and training of the large-language model.
Data quality analysis
Analysis and improvement of data quality.
Model fine tuning
Adapting the pre-trained large language model (BERT) to the requirements of health insurance.
Automation
Automate categorization and sentiment analysis of feedback to optimize operational processes.
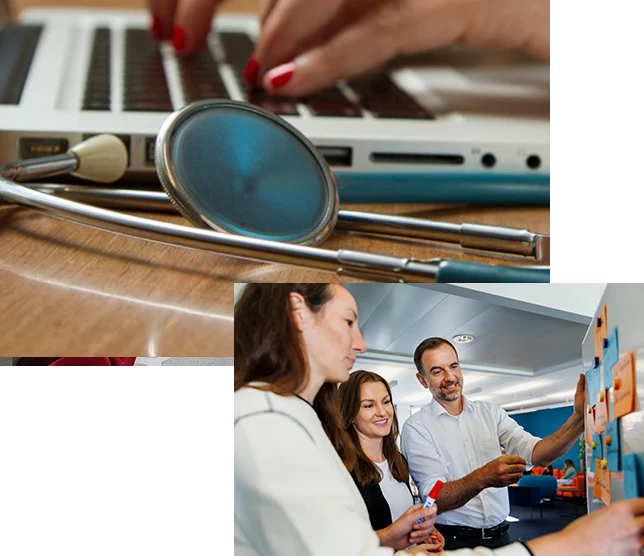
Results & Successes
Consistent quality: The automated categorization of customer feedback ensures more consistent and reproducible results than manual methods.
Extensible automation: The solution enables expandable automation of feedback categorization and can be used in other areas of customer service.
Reduction of operating costs: The integration of the model into business processes ensures shorter response times and faster control of internal processes and thus to operational cost reductions.
The project involves development and implementation of methods to categorize customer feedback automatically at a consistently high constant quality. The model already enables extensible automation of the categorization of a large part of the feedback. It can also be used to check and improve data quality. After integration into business processes, it thus becomes possible to guarantee faster and more consistent control of internal processes in addition to faster responses for customers. As a result, data quality can be improved and operational costs can be reduced. The model can be extended to full automation and other languages, and also used in other areas of customer service.
The Tech Behind the Success

Download the Full Story
Want a handy PDF version of our success story? Whether you need it for yourself or to introduce the project to your team, download it now and explore the full success story. Enjoy reading!
Inspired?
Did our success stories spark your interest? If you're facing similar challenges in data, analytics and AI and look for expert support, let’s talk. A brief call can reveal how we can help you move forward.
