
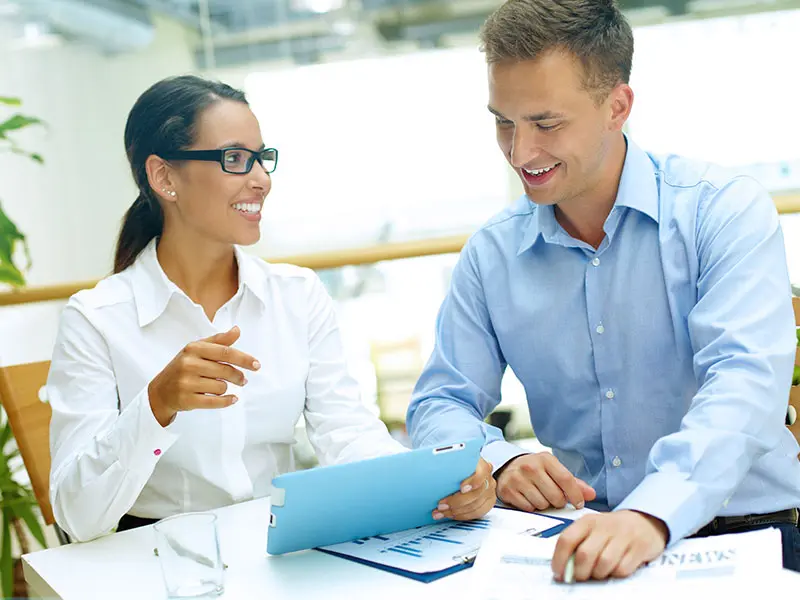
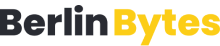
Siemens Financial Services: The Financial Data Platform of the Future
Data-driven potential as a success factor for the financial sector
Initial Situation & Challenge
Siemens Financial Services GmbH (SFS) is an international provider of financial solutions in the B2B sector. A homogeneous data landscape is of great importance to the company to ensure quick and reliable decision-making, enhance efficiency, and reduce costs. Additionally, real-time analytics and high data quality are crucial to keeping up with competitors in the fast-paced financial world. However, in early 2022, Siemens Financial Services faced a rather fragmented data landscape in the Data & Analytics domain.
Based on an internally developed data strategy, it was determined that the establishment of a dedicated SFS data platform would be the objective. Siemens Financial Services therefore engaged the experts at b.telligent to ensure long-term stability and competitiveness through a new platform. This platform aimed to reduce complexity and serve as a central, reliable data source (“Single Source of Truth”). The project focused on two primary challenges: first, the immense workload, as it required integrating up to 30 individual source systems—often with several hundred source tables. Second, the regulatory requirements in the financial sector, particularly concerning data security, data protection, access permissions, and governance.
Solution
A "classic" solution was quickly ruled out by the project team due to the enormous workload. Instead, an early decision was made in collaboration with the client to adopt a metadata-driven approach, which also influenced the selection of components.
The new (primary) cloud platform chosen was Microsoft Azure, which could be ideally extended with b.telligent’s metaFactory. This not only allows for the automated ingestion of data sources into the Azure Data Lake (ADLS Gen2) but also analyzes these sources and adds extracted information to the metadata. The parameterized Synapse Pipelines enable processing up to the Historized Layer (Delta Parquet), where the source data is versioned and made available for further use.
Automated Processing and Streaming Architecture
Next, the b.telligent team focused on automated processing in the Snowflake Data Warehouse using Data Vault methodology for the Core Layer. To ensure seamless integration, they extended the b.telligent metaFactory with a generator for Snowflake External Tables. With this consistent ELT approach, automation with Datavault Builder was conducted exclusively within Snowflake. While metadata usage allowed for the automation of many processing steps, some development effort was still required.
Certain reporting requirements necessitated the establishment of a streaming architecture to provide users with timely and up-to-date data in Snowflake. Data originating from a PostgreSQL database was prepared for streaming using Debezium and written into a local Kafka cluster. Mirror Maker was then used to replicate the data from one Kafka cluster to another, enabling replication to the cloud via an Azure Event Hub, which subsequently stored the data in the Azure Data Lake via Event Capture. The interplay between EventGrid and Storage Queue allowed for the efficient transfer of data to Snowflake for further processing.
It quickly became evident that this area also needed to be automated as efficiently as possible. The approach included Continuous Integration/Continuous Deployment (CI/CD) via Azure DevOps, a carefully planned release management process, and the provision of independent development environments (sandboxes) for each use case. At peak times, up to 90 developers and 200 business testers worldwide were working on the project.
Secure Authorization Management for Regulatory Requirements
To address regulatory requirements from the outset, a range of functional roles for users (developers and consumers) were defined. These roles could be automatically mapped to technical roles and assigned to corresponding user accounts in applications such as Snowflake, Azure, and Datavault Builder via the central user provisioning system within Siemens. Additionally, in Snowflake, Row- and Column-Level Policies were automatically implemented to enforce the "Need-to-Know" principle, which is crucial in the financial industry. Individual permissions could then be requested via self-service and workflow within the user provisioning system.

b.metaFactory
The b.telligent metaFactory is a powerful framework developed by b.telligent for data integration with Azure Data Factory. With over 100 connectors, including those for Oracle and SAP, it offers maximum flexibility in connecting various source systems. Parameterized pipelines and a central control table allow for the easy and quick integration of new data sources. Data is stored in a high-performance Delta format in the Azure Data Lake. Thanks to comprehensive logging and monitoring functions, the metaFactory ensures the highest levels of maintainability and security—all within the Azure Subscription. Fast, secure, and future-proof: your data, optimally integrated.
Voices From the Project
b.telligent Services at a Glance
Metadata-Driven Approach
Utilization of b.telligent metaFactory for automated data ingestion and analysis in Azure Data Lake
Seamless Integration
Implementation of Snowflake as a Data Warehouse for efficient data processing and regulatory compliance
Automation and Efficiency
Use of Datavault Builder for DWH automation, combined with CI/CD pipelines and flexible development environments
Self-Service Permissions
Integration of all components into a user-friendly workflow for access requests via a user provisioning system
Real-Time Architecture
Streaming architecture with Debezium, Kafka, Azure Event Hub, and Snowflake for real-time data availability and efficient reporting
Regulatory Compliance
Definition of functional and technical roles, along with automated access policies in Snowflake
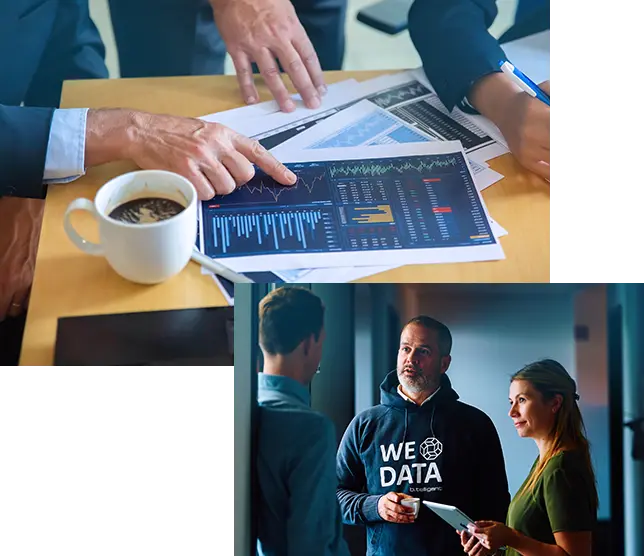
Results & Successes
Automated data integration: With the b.telligent metaFactory, over 20 source systems were efficiently integrated into the new Financial Data Platform.
High scalability & performance: The combination of Microsoft Azure and Snowflake enables real-time analyses and parallel developments thanks to sandbox architecture.
Regulatory security: Automated authorization management ensures compliance according to the need-to-know principle.
Thanks to the consistent automation of data integration through metadata and a well-thought-out release management process, the b.telligent team successfully integrated data from more than 20 source systems into the new SFS data platform within just 24 months. A key success factor was the chosen sandbox architecture, which minimized dependencies and interactions between development streams.
By investing in a tool for the automated migration of SAP HANA Calculation Views to standard SQL, existing reports in the presentation layer could continue to be used. Additionally, easier data access now provides the client with new opportunities in the fields of Data Science and Machine Learning.
While it was clear that replacing SAP HANA with the new platform would not immediately result in cost savings, the long-term benefits and future-proofing of the solution more than compensate for this. Moreover, the integration of all components into the central user provisioning system significantly accelerated the onboarding of new users.
The Tech Behind the Success
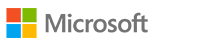
Microsoft
Innovation and integration, as well as interoperability, are key factors of the Microsoft product development. Learn more about our collaboration!
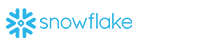
Snowflake
b.telligent builds high-performance and scalable analytics solutions in the cloud with Snowflake. Learn more about our partnership now!

Datavault Builder
Datavault Builder's efficient visual data integration solution focuses on standardization and collaboration between business and IT staff. This allows you to increase productivity and benefit from the fastest possible time-to-insights, with full auditability and governance without additional effort.

Download the Full Story
Want a handy PDF version of our success story? Whether you need it for yourself or to introduce the project to your team, download it now and explore the full success story. Enjoy reading!
Inspired?
Did our success stories spark your interest? If you're facing similar challenges in data, analytics and AI and look for expert support, let’s talk. A brief call can reveal how we can help you move forward.
